Application Example - ALS Classification
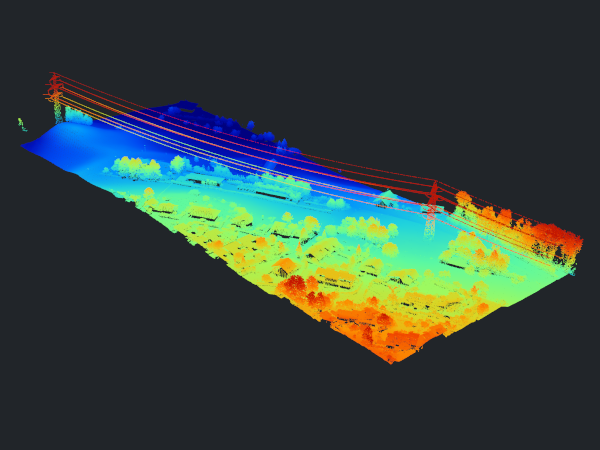
1) Manage and import data
Import your data for processing.
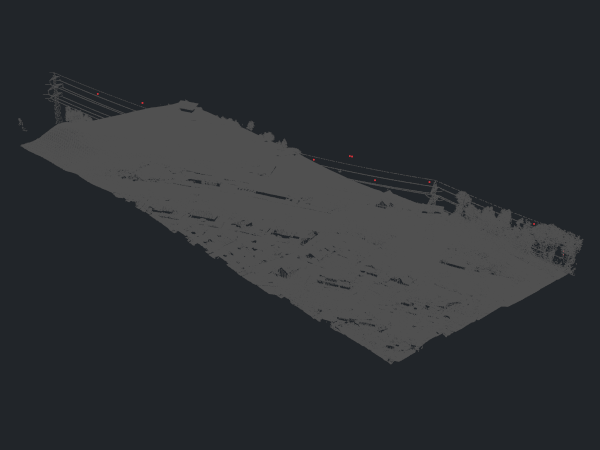
Python Code
import os
import project_config as cfg
from PySAGA import saga_api, lis_cmd
from PySAGA.tools import lis_filtering
# -----------------------------------------------------------------------------
# filter noise points
lis_filtering.Remove_Isolated_Points(PC_IN=pc_buff, COPY_ATTR=True, SEARCH_RADIUS=3,
MAX_POINTS=2, METHOD='label points', ATTR_NAME='noise', CLASSID=7,
CLASSID_UNDEF=1)
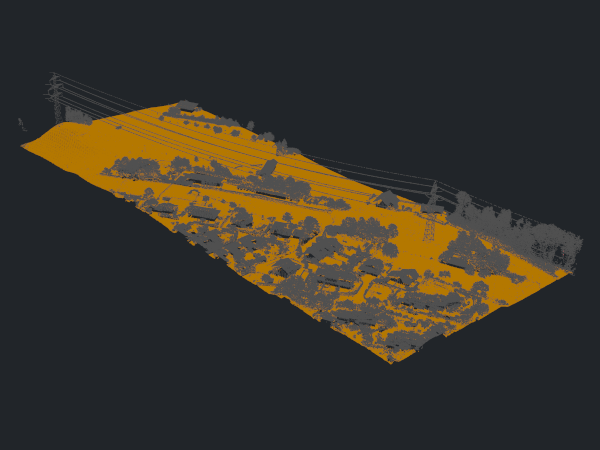
Python Code
import os
import project_config as cfg
from PySAGA import saga_api, lis_cmd
from PySAGA.tools import lis_classification
# -----------------------------------------------------------------------------
# ground non-ground classification
lis_classification.Ground_Classification(PC_IN=pc_buff, FIELD_CLASS='noise', COPY_ATTR=True,
INIT_CELLSIZE=50, FINAL_CELLSIZE=0.5,
FILTER_SEEDS=False, TERRAIN_ANGLE=88, MIN_EDGE_LENGTH=0.5,
MAX_ANGLE=35, MAX_DISTANCE=0.8,
Z_TOLERANCE=0.05, ATTACH_DZ=True, EXCLUDE_CLASSES='7')
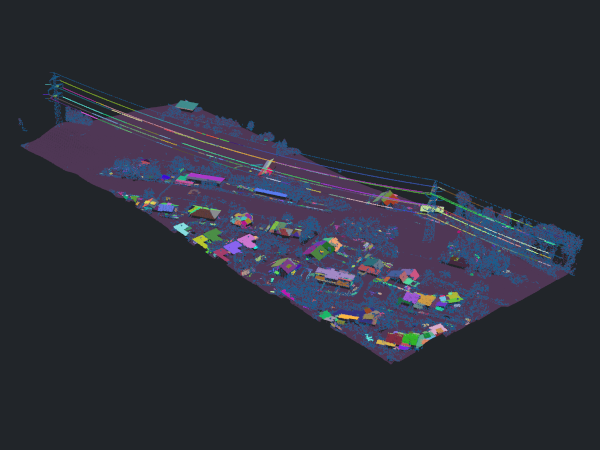
4) Find planar patches
Find planar patches such as building roofs to prepare for building and vegetation classification.
Python Code
import os
import project_config as cfg
from PySAGA import saga_api, lis_cmd
from PySAGA.tools import lis_segmentation
# -----------------------------------------------------------------------------
# find planar patches
lis_segmentation.Segmentation_by_Plane_Growing(PC_IN=pc_buff, FILTER_FIELD='grdclass', COPY_ATTR=True, LOW_RANGE='0;1',
HIGH_RANGE='3;64', METHOD_NN='radius', RADIUS_NN=1,
MIN_K=3, ROBUST=True, ROBUST_THRES=0.1, ROBUST_PERCENTAGE=50.0,
METHOD_ROBUST_SEARCH_POINT='search point must be included in best fit plane',
GROWING_RADIUS=0.5, GROWING_PLANE_DIST=0.1,
SEG_ITERATIONS=1, REGION_RADIUS=1, REGION_MINSIZE=20,
REGION_MAXSIZE=100000, REGION_MAXANGLE=10, REGION_MAXOFFSET=0.2,
REGION_RECALC_NORMAL=100000)
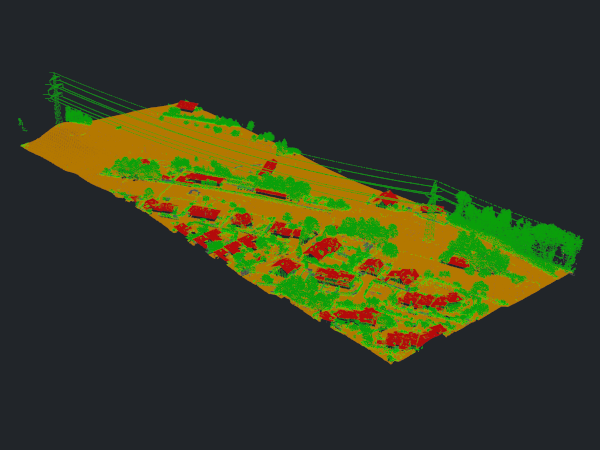
5) Classify buildings
Classify your data into ground, buildings and vegetation points.
Python Code
import os
import project_config as cfg
from PySAGA import saga_api, lis_cmd
from PySAGA.tools import lis_classification
# -----------------------------------------------------------------------------
# enhanced classification
lis_classification.Enhanced_Point_Cloud_Classification(PC_IN=pc_buff, FIELD_SEGMENTID='segmentid', FIELD_CLASSID_GROUND='grdclass',
FIELD_DZ='dz', COPY_ATTR=True, EXCLUDE_CLASSES='7', METHOD_BUILDING='point cloud features',
MIN_DZ_BUILDING=2.0, GROWING_RADIUS_BUILDING=1,
FEATURE_RADIUS_BUILDING=1, MIN_NEIGHBOR_RATIO_BUILDING=60.0,
SLOPE_ADAPTIVE_RATIO=False, MIN_POINTS_BUILDING=200, RADIUS_VEGETATION=2.0,
MIN_POINTS_VEGETATION=5, MIN_DZ_LOW_VEGETATION=0, MIN_DZ_MEDIUM_VEGETATION=0.3,
MIN_DZ_HIGH_VEGETATION=2, OUTPUT_CALIBRATION=False)
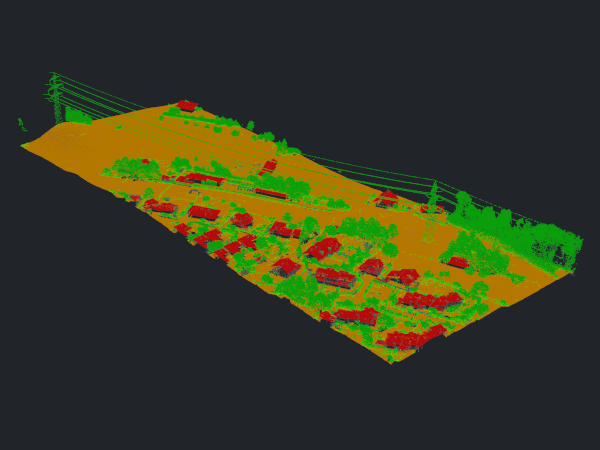
6) Clean building roofs and facades
Clean up your building classification by relabeling incorrect classifications on roofs and facades.
Python Code
import os
import project_config as cfg
from PySAGA import saga_api, lis_cmd
from PySAGA.tools import lis_classification
# -----------------------------------------------------------------------------
# clean classification
lis_classification.Clean_Building_Facades(PC_IN=pc_buff, FIELD_FILTERATTR='classid', COPY_ATTR=True, ATTR_SUFFIX='facades',
RADIUS_NN=1, BUILDING_CLASS='6;6', LOW_ATTR_RANGE='3;5',
HIGH_ATTR_RANGE='7;64', TARGET_CLASS=1)
lis_classification.Clean_Building_Roofs(PC_IN=pc_buff, FIELD_FILTERATTR='classid_facades', COPY_ATTR=True, ATTR_SUFFIX='roofs',
DIMENSION='3D search', RADIUS_NN=2.5, USE_INNER_RADIUS=False, THRES_MAJORITY=25,
TARGET_CLASS=1, FILTER_FACADE=False)
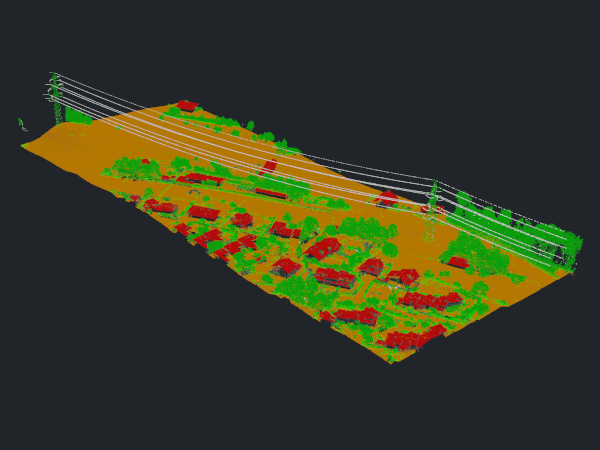
7) Classify powerlines
Classify your data into ground, buildings, vegetation and powerlines.
Python Code
import os
import project_config as cfg
from PySAGA import saga_api, lis_cmd
from PySAGA.tools import lis_classification
# -----------------------------------------------------------------------------
# powerline classification
lis_classification.Power_Line_Classification(PC_IN=pc_buff, FIELD_CLASS='classid_facades_roofs', FIELD_THRES='dz',
COPY_ATTR=True, ATTR_SUFFIX='pl', EXCLUDE_CLASSES='7', THRES_RANGE='3;1000',
SEARCH_RADIUS=5, CLASSIFY_POLES=False)
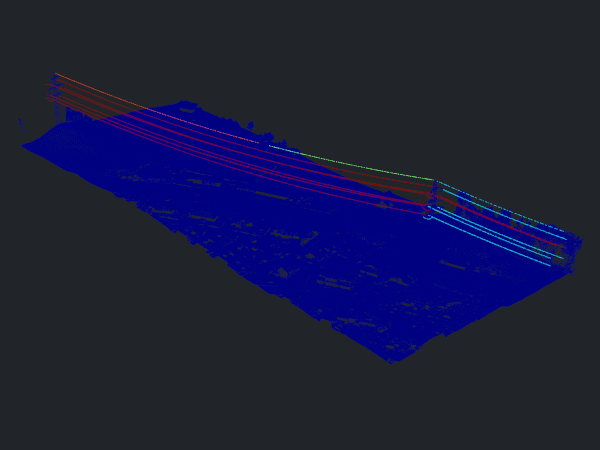
8) Minimum powerline length
Define the minimum length of the derived powerline objects for filtering.
Python Code
import os
import project_config as cfg
from PySAGA import saga_api, lis_cmd
from PySAGA.tools import lis_segmentation
from PySAGA.tools import lis_analysis
# -----------------------------------------------------------------------------
# powerline classification
lis_segmentation.Densitybased_Spatial_Clustering(PC_IN=pc_buff, FIELD_ATTR_1='X', FIELD_ATTR_2='Y', FIELD_ATTR_3='Z',
FIELD_CONSTRAINT='classid_pl', COPY_ATTR=True, ATTR_SUFFIX='pl',
CHOICE_DIM='3-dimensional', SEARCH_RADIUS=2, MIN_POINTS=1,
RANGE_CONSTRAINT='14;14', TOL_CONSTRAINT='-0.5;0.5')
lis_analysis.Segment_Features(PC_IN=pc_buff, FIELD_SEGMENTID='cluster_pl', COPY_ATTR=True, LENGTH_2D=True)
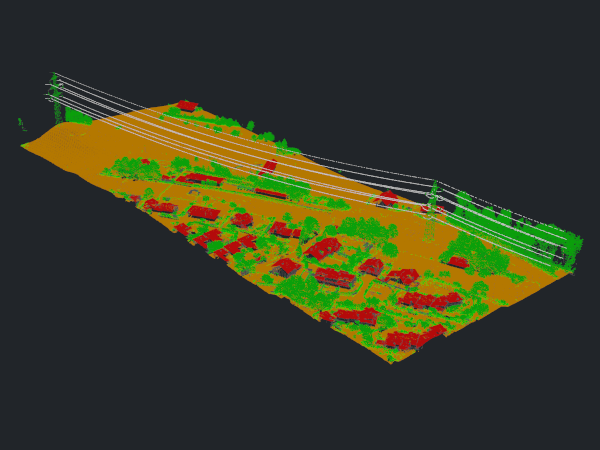
9) Filter powerlines
Clean up the powerline classification based on the defined minimum object length.
Python Code
import os
import project_config as cfg
from PySAGA import saga_api, lis_cmd
from PySAGA.tools import lis_filtering
from PySAGA.tools import lis_arithmetic
# -----------------------------------------------------------------------------
# powerline filtering
lis_filtering.Attribute_Filter(PC_IN=pc_buff, FIELD_ATTR_1='length2d', COPY_ATTR=True, METHOD='binary flag',
TYPE_1='lower limit (min)', MIN_1=5, ATTR_NAME='pl_filtered')
lis_arithmetic.Point_Cloud_Attribute_Calculator(PC_IN=pc_buff,
FORMULA='ifelse(eq([classid_pl],14),ifelse(eq([pl_filtered],1),14,1),[classid_pl])',
NAME='classid_pl_filtered', TYPE='1 byte unsigned integer',
INCLUDE_NODATA=False)
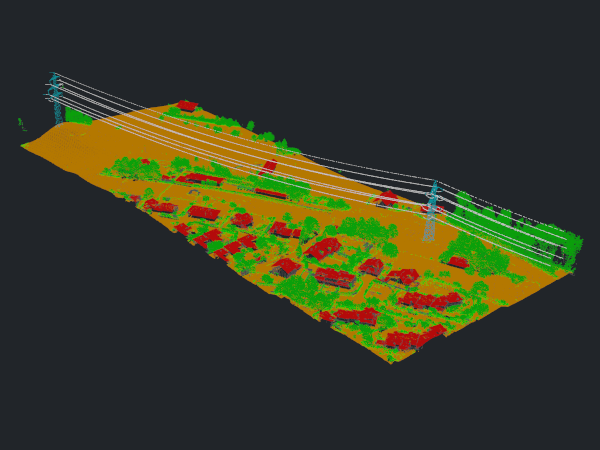
Python Code
import os
import project_config as cfg
from PySAGA import saga_api, lis_cmd
from PySAGA.tools import lis_classification
# -----------------------------------------------------------------------------
# pylon classification
lis_classification.Pylon_Classification(PC_IN=pc_buff, FIELD_CLASS='classid_pl_filtered', FIELD_DZ='dz',
COPY_ATTR=True, ATTR_SUFFIX='pylon', MIN_PYLON_HEIGHT=20,
MAX_PYLON_DIAMETER=25.0, RADIUS_NN=3.0, MAX_DIFFERENCE=1.0, MIN_POINTS=200,
SEED_CLASS=14, EXCLUDE_CLASSES='7', OUTPUT_CALIBRATION=False)
Complete Python Example Script
import os
import project_config as cfg
from PySAGA import saga_api, lis_cmd
from PySAGA.tools import lis_virtual
from PySAGA.tools import lis_filtering
from PySAGA.tools import lis_classification
from PySAGA.tools import lis_segmentation
from PySAGA.tools import lis_analysis
from PySAGA.tools import lis_arithmetic
from PySAGA.tools import lis_tools
SEARCH_RADIUS_OUTLIER = 3.0
TILE_OVERLAP = 30.0
GRD_INITIAL_CELLSIZE = 50.0
GRD_FINAL_CELLSIZE = 0.5
GRD_MAX_ANGLE = 35.0
GRD_MAX_DISTANCE = 0.8
SEARCH_RADIUS = 1.0
SEARCH_RADIUS_PL = 5.0
MIN_WIRE_LENGTH = 5.0
MIN_PYLON_HEIGHT = 20.0
@lis_cmd.proc_function_wrapper
def Task(TaskNum=0):
# -----------------------------------------------------------------------------
# load the shapefile with the tiling scheme used for processing
tileshape = saga_api.SG_Get_Data_Manager().Add_Shapes('PathTileshape/Tiles.shp')
if not tileshape.is_Valid():
return lis_cmd.Error_Return('Loading tileshape ' + 'PathTileshape/Tiles.shp' + ' failed')
# -----------------------------------------------------------------------------
# get tile to process
tile = tileshape.Get_Shape(TaskNum)
xmin = tile.Get_Extent().Get_XMin()
ymin = tile.Get_Extent().Get_YMin()
xmax = tile.Get_Extent().Get_XMax()
ymax = tile.Get_Extent().Get_YMax()
# tiles are referenced by their lower-left corner coordinate
lis_cmd.Message_Print('\nProcessing tile {:.0f}_{:.0f} \n'.format(xmin, ymin))
tilename = str(int(xmin)) + '_' + str(int(ymin))
# -----------------------------------------------------------------------------
# start processing pipeline
# -----------------------------------------------------------------------------
# query point cloud (with overlap) from virtual point cloud dataset
pc_out_list = []
if not lis_virtual.Get_Subset_from_Virtual_LASLAZ(PC_OUT=pc_out_list, FILENAME=cfg.LASVF, COPY_ATTR=True,
CONSTRAIN_QUERY=False, FILE_COMPRESSION=True, ERROR_IF_EMPTY=True,
THIN_OUT=True, SPACING=0.01, AOI_XRANGE=str(xmin)+';'+str(xmax),
AOI_YRANGE=str(ymin)+';'+str(ymax), AOI_ADD_OVERLAP=True,
OVERLAP=TILE_OVERLAP, SKIP_EMPTY_AOIS=True,
METHOD_CLIP='lower and left boundary is inside'):
return lis_cmd.Error_Return('Failed to execute tool on tile ' + tilename)
if len(pc_out_list) < 1:
return lis_cmd.Error_Return('Tile ' + tilename + ' contains no point cloud data, skipping!')
# add the point cloud datasets to the data manager for easier memory management
pc_buff = saga_api.SG_Get_Data_Manager().Add(pc_out_list[0]).asPointCloud()
if pc_buff.Get_Count() < 5:
return lis_cmd.Error_Return('Tile ' + tilename + ' has less than 5 points, skipping ...')
# -----------------------------------------------------------------------------
# filter noise points
if not lis_filtering.Remove_Isolated_Points(PC_IN=pc_buff, COPY_ATTR=True, SEARCH_RADIUS=SEARCH_RADIUS_OUTLIER,
MAX_POINTS=2, METHOD='label points', ATTR_NAME='noise', CLASSID=7,
CLASSID_UNDEF=1):
return lis_cmd.Error_Return('Failed to execute tool on tile ' + tilename)
# -----------------------------------------------------------------------------
# ground classification
if not lis_classification.Ground_Classification(PC_IN=pc_buff, FIELD_CLASS='noise', COPY_ATTR=True,
INIT_CELLSIZE=GRD_INITIAL_CELLSIZE, FINAL_CELLSIZE=GRD_FINAL_CELLSIZE,
FILTER_SEEDS=False, TERRAIN_ANGLE=88, MIN_EDGE_LENGTH=GRD_FINAL_CELLSIZE,
MAX_ANGLE=GRD_MAX_ANGLE, MAX_DISTANCE=GRD_MAX_DISTANCE,
Z_TOLERANCE=0.05, ATTACH_DZ=True, EXCLUDE_CLASSES='7'):
return lis_cmd.Error_Return('Failed to execute tool on tile ' + tilename)
# -----------------------------------------------------------------------------
# segmentation of planar objects for building roof detection
if not lis_segmentation.Segmentation_by_Plane_Growing(PC_IN=pc_buff, FILTER_FIELD='grdclass', COPY_ATTR=True, LOW_RANGE='0;1',
HIGH_RANGE='3;64', METHOD_NN='radius', RADIUS_NN=SEARCH_RADIUS,
MIN_K=3, ROBUST=True, ROBUST_THRES=0.1, ROBUST_PERCENTAGE=50.0,
METHOD_ROBUST_SEARCH_POINT='search point must be included in best fit plane',
GROWING_RADIUS=SEARCH_RADIUS / 2.0, GROWING_PLANE_DIST=0.1,
SEG_ITERATIONS=1, REGION_RADIUS=SEARCH_RADIUS, REGION_MINSIZE=20,
REGION_MAXSIZE=100000, REGION_MAXANGLE=10, REGION_MAXOFFSET=0.2,
REGION_RECALC_NORMAL=100000):
return lis_cmd.Error_Return('Failed to execute tool on tile ' + tilename)
# -----------------------------------------------------------------------------
# building and vegetation classification
if not lis_classification.Enhanced_Point_Cloud_Classification(PC_IN=pc_buff, FIELD_SEGMENTID='segmentid', FIELD_CLASSID_GROUND='grdclass',
FIELD_DZ='dz', COPY_ATTR=True, EXCLUDE_CLASSES='7', METHOD_BUILDING='point cloud features',
MIN_DZ_BUILDING=2.0, GROWING_RADIUS_BUILDING=SEARCH_RADIUS,
FEATURE_RADIUS_BUILDING=SEARCH_RADIUS, MIN_NEIGHBOR_RATIO_BUILDING=60.0,
SLOPE_ADAPTIVE_RATIO=False, MIN_POINTS_BUILDING=200, RADIUS_VEGETATION=SEARCH_RADIUS * 2.0,
MIN_POINTS_VEGETATION=5, MIN_DZ_LOW_VEGETATION=0, MIN_DZ_MEDIUM_VEGETATION=0.3,
MIN_DZ_HIGH_VEGETATION=2, OUTPUT_CALIBRATION=False):
return lis_cmd.Error_Return('Failed to execute tool on tile ' + tilename)
# -----------------------------------------------------------------------------
# clean building facades
if not lis_classification.Clean_Building_Facades(PC_IN=pc_buff, FIELD_FILTERATTR='classid', COPY_ATTR=True, ATTR_SUFFIX='facades',
RADIUS_NN=SEARCH_RADIUS, BUILDING_CLASS='6;6', LOW_ATTR_RANGE='3;5',
HIGH_ATTR_RANGE='7;64', TARGET_CLASS=1):
return lis_cmd.Error_Return('Failed to execute tool on tile ' + tilename)
# -----------------------------------------------------------------------------
# clean building roofs
if not lis_classification.Clean_Building_Roofs(PC_IN=pc_buff, FIELD_FILTERATTR='classid_facades', COPY_ATTR=True, ATTR_SUFFIX='roofs',
DIMENSION='3D search', RADIUS_NN=2.5, USE_INNER_RADIUS=False, THRES_MAJORITY=25,
TARGET_CLASS=1, FILTER_FACADE=False):
return lis_cmd.Error_Return('Failed to execute tool on tile ' + tilename)
# -----------------------------------------------------------------------------
# power line classification
if not lis_classification.Power_Line_Classification(PC_IN=pc_buff, FIELD_CLASS='classid_facades_roofs', FIELD_THRES='dz',
COPY_ATTR=True, ATTR_SUFFIX='pl', EXCLUDE_CLASSES='7', THRES_RANGE='3;1000',
SEARCH_RADIUS=SEARCH_RADIUS_PL, CLASSIFY_POLES=False):
return lis_cmd.Error_Return('Failed to execute tool on tile ' + tilename)
# -----------------------------------------------------------------------------
# clustering of power line points for filtering
if not lis_segmentation.Densitybased_Spatial_Clustering(PC_IN=pc_buff, FIELD_ATTR_1='X', FIELD_ATTR_2='Y', FIELD_ATTR_3='Z',
FIELD_CONSTRAINT='classid_pl', COPY_ATTR=True, ATTR_SUFFIX='pl',
CHOICE_DIM='3-dimensional', SEARCH_RADIUS=SEARCH_RADIUS * 2, MIN_POINTS=1,
RANGE_CONSTRAINT='14;14', TOL_CONSTRAINT='-0.5;0.5'):
return lis_cmd.Error_Return('Failed to execute tool on tile ' + tilename)
# -----------------------------------------------------------------------------
# calculate power line cluster lengths for filtering
if not lis_analysis.Segment_Features(PC_IN=pc_buff, FIELD_SEGMENTID='cluster_pl', COPY_ATTR=True, LENGTH_2D=True):
return lis_cmd.Error_Return('Failed to execute tool on tile ' + tilename)
# -----------------------------------------------------------------------------
# filter power line clusters by minimum length
if not lis_filtering.Attribute_Filter(PC_IN=pc_buff, FIELD_ATTR_1='length2d', COPY_ATTR=True, METHOD='binary flag',
TYPE_1='lower limit (min)', MIN_1=MIN_WIRE_LENGTH, ATTR_NAME='pl_filtered'):
return lis_cmd.Error_Return('Failed to execute tool on tile ' + tilename)
# -----------------------------------------------------------------------------
# relabel power line classification
if not lis_arithmetic.Point_Cloud_Attribute_Calculator(PC_IN=pc_buff,
FORMULA='ifelse(eq([classid_pl],14),ifelse(eq([pl_filtered],1),14,1),[classid_pl])',
NAME='classid_pl_filtered', TYPE='1 byte unsigned integer',
INCLUDE_NODATA=False):
return lis_cmd.Error_Return('Failed to execute tool on tile ' + tilename)
# -----------------------------------------------------------------------------
# pylon classification
if not lis_classification.Pylon_Classification(PC_IN=pc_buff, FIELD_CLASS='classid_pl_filtered', FIELD_DZ='dz',
COPY_ATTR=True, ATTR_SUFFIX='pylon', MIN_PYLON_HEIGHT=MIN_PYLON_HEIGHT,
MAX_PYLON_DIAMETER=25.0, RADIUS_NN=3.0, MAX_DIFFERENCE=1.0, MIN_POINTS=200,
SEED_CLASS=14, EXCLUDE_CLASSES='7', OUTPUT_CALIBRATION=False):
return lis_cmd.Error_Return('Failed to execute tool on tile ' + tilename)
# -----------------------------------------------------------------------------
# remove tile overlap
pc_out = saga_api.SG_Get_Data_Manager().Add_PointCloud()
if not lis_tools.Clip_Point_Cloud_with_Extent(PC_IN=pc_buff, PC_OUT=pc_out,
X_EXTENT=str(xmin)+';'+str(xmax), Y_EXTENT=str(ymin)+';'+str(ymax),
METHOD_CLIP='lower and left boundary is inside'):
return lis_cmd.Error_Return('Failed to execute tool on tile ' + tilename)
# -----------------------------------------------------------------------------
# save classified tile (without overlap)
pc_out.Save('YourOutputPath' + os.sep + 'tile_' + tilename + '.sg-pts-z')
# -----------------------------------------------------------------------------
# free memory resources and return success
saga_api.SG_Get_Data_Manager().Delete()
return True
# -----------------------------------------------------------------------------
# -----------------------------------------------------------------------------
# -----------------------------------------------------------------------------
# the main function
#
if __name__ == '__main__':
# -----------------------------------------------------------------------------
# start the logging
starttime = lis_cmd.Start_Logging(False)
# -----------------------------------------------------------------------------
# load the shapefile with the tiling scheme used for processing
tiles = saga_api.SG_Get_Data_Manager().Add_Shapes('PathTileshape/Tiles.shp')
if not tiles.is_Valid():
lis_cmd.Error_Exit('Loading tileshape ' + 'PathTileshape/Tiles.shp' + ' failed', starttime, True)
# -----------------------------------------------------------------------------
# process the Task function in parallel (each tile on a CPU core) with the maximum number of available CPU cores
lis_cmd.Run_Parallel_Shapes(Task, tiles, saga_api.SG_OMP_Get_Max_Num_Threads())
# -----------------------------------------------------------------------------
# parallelized processing completed, now ...
# create virtual point cloud dataset of classified tiles for subsequent processing steps (here LAS/LAZ export in script #3)
inputfiles = lis_cmd.Create_File_List('YourOutputPath', '.sg-pts;.sg-pts-z', 'YourOutputPath' + os.sep + 'spcvf_input_file_list.txt')
if not lis_virtual.Create_Virtual_Point_Cloud_Dataset(INPUT_FILE_LIST=inputfiles, FILENAME='YourOutputPath' + os.sep + 'tiles.spcvf',
METHOD_PATHS=1, USE_HEADER=True):
lis_cmd.Error_Exit('Failed to execute tool', starttime, True)
# -----------------------------------------------------------------------------
# free memory and stop logging
saga_api.SG_Get_Data_Manager().Delete()
lis_cmd.Stop_Logging(starttime)